Protecting our online spaces has become essential in a time when the digital world permeates every part of our lives. AI has become a potent ally in the cybersecurity field due to the impending threat of cyberattacks, which calls for sophisticated technologies. AI actively anticipates and neutralizes emerging cyber threats and fortifies defenses through state-of-the-art algorithms and machine learning. This investigation explores the area where cybersecurity and AI converge and where current research influences the direction of digital defense.
Introduction to AI in Cybersecurity
It’s essential to grasp the fundamental ideas before exploring AI’s role in protecting digital spaces. AI research aims to improve robots’ capacity for autonomous decision-making, learning, and adaptability. This cybersecurity framework provides the foundation for automated systems that guarantee fast and accurate real-time cyber threat detection, analysis, and response. Current research indicates that a great deal of work has been made in creating AI models that can identify complex patterns in massive datasets, making it possible to identify anomalies that could be signs of security breaches quickly. To demonstrate the dynamic evolution of AI in defending digital gateways in the face of a constantly shifting threat landscape, ongoing research endeavors to improve AI algorithms’ capacity to anticipate and mitigate new and recognized hazards.
Machine Learning in Cybersecurity
Machine learning is unquestionably a foundational element as we explore the complex levels of artificial intelligence in cybersecurity. Through experience-based learning, this branch of artificial intelligence enables systems to change and adapt without explicit programming. When it comes to cybersecurity, machine learning algorithms are essential for bolstering our defenses against a wide range of online dangers.
Detecting and Preventing Cyber Attacks
Machine learning algorithms are essential tools in the ever-changing field of cybersecurity because they can identify trends and abnormalities in large datasets. These intrinsic abilities play an integral part in strengthening digital defenses and proactively detecting possible cyber threats before they have a chance to cause damage. Let’s dissect the intricate mechanisms of machine learning to enhance cybersecurity defenses.
Understanding the Mechanism
- Feature Extraction
- Data Representation: The first step in the process is representing the data, where machine learning methods highlight relevant elements such as user behaviors, system events, and network traffic.
- Dimensionality reduction: This strategy simplifies complex datasets by emphasizing essential elements that improve the algorithms’ capacity to recognize significant patterns.
- Training the model
- Supervised Learning: Several cybersecurity machine learning algorithms use a supervised learning paradigm. The algorithm is fed labelled data during training, defining malicious and typical activity instances.
- Building a Decision Boundary: Given the labelled data, the algorithm creates a decision boundary separating regular activity from anomalous behavior. This boundary serves as the basis for later real-time anomaly identification.
- Real-time Analysis
- Continuous Monitoring: The machine learning model is deployed post-training and monitors incoming data, such as system logs, network traffic, and user interactions, in real-time.
- Anomaly Detection: The model flags possible anomalies and initiates automated reactions or alerts when it comes across fresh data by comparing patterns against the predetermined decision boundary.
- Adaptation and Learning
- Dynamic Updating: Machine learning models are updated dynamically to address new threats and maintain adaptability to shifting cyber threat environments.
Real world Applications
Advances in machine learning have led to innovative cybersecurity solutions that are transforming defenses against a constantly changing array of threats. Now let’s explore a few real-world instances that demonstrate the observable benefits of machine learning algorithms.
- Malware Detection
Example: Safeguarding Critical Infrastructure- Antivirus solutions are surpassed by machine learning in their ability to detect and neutralize zero-day malware.
- Responding quickly and strengthening defenses was possible since an advanced model correctly predicted the appearance of a zero-day variant.
- Anomaly Detection
Example: Safeguarding Critical Infrastructure- Critical infrastructure is protected by machine learning’s abnormality detection skills, which quickly detect deviations from baseline patterns.
- Investigating and reducing potential cyber-attacks that target critical systems proactively is made possible by real-time detection.
- Phishing Detection
Example: Mitigating Complex Phishing Attacks- A key component of countering sophisticated phishing attacks is machine learning.
- The model detects minor anomalies in user behavior, content, and email trends, enabling cybersecurity professionals to quickly stop data breaches and foil phishing efforts.
Behavioral Analytics and Anomaly Detection
Behavioral analytics and potent AI agents in cybersecurity combine to provide a formidable defense against cyberattacks. This dynamic combination challenges conventional security procedures by identifying distinct patterns in user behavior and network activity. When combined, AI and behavioral analytics are the best at identifying, foreseeing, and foiling cyberattacks, raising the bar for modern security.
- Discovering Behavioral Analytics
Behavioral analytics is a critical component of modern cybersecurity, analyzing network traffic, system actions, and user behavior to establish a baseline of consistent activity. This proactive discipline serves as a defense against intrusions by promptly identifying irregularities. Through comprehensive digital ecosystem analysis, behavioral analytics shows “normal” behavior. It uses that understanding to deliver early warnings, facilitate preventative action, and function as a trustworthy manual for effective cyber vigilance. - AI Agents as Perceptive Guardians
AI agents, who serve as sentinel guardians with cutting-edge algorithms and machine learning capabilities, are essential to anomaly detection. In this domain, network traffic monitoring and user behavior analysis function as the carefully crafted orchestra these vigilant protectors utilize to reveal possible cyber threats.- Precision in User Behavior Analysis
AI agents excel at analyzing user activity, swiftly identifying anomalies such as unusual login times or data requests. This accuracy enhances overall security by rapidly detecting compromised accounts and insider threats, ensuring proactive security measures. - Network Traffic Monitoring
AI agents act as perceptive managers, constantly monitoring network flow for irregularities. By establishing a baseline of normal behavior, they quickly detect anomalies like sudden data spikes, preventing potential dangers through real-time data analysis. Predictive techniques ensure prompt identification and resolution of vulnerabilities. - Real-Time Anomaly Detection
In cybersecurity, AI agents, and behavioral analytics collaborate for proactive defense. Through adaptive learning, these agents recognize aberrant behavior amidst shifting dynamics, responding with alarms, automated security processes, or isolating infected accounts. This proactive approach prevents data breaches and limits the impact of illegal access.
- Precision in User Behavior Analysis
Automated Threat Detection and Response
AI has transformed cybersecurity with automated threat detection and response, drastically reducing the time to eliminate cyberattacks. Leveraging real-time dataset analytics and complex algorithms, AI swiftly identifies trends, expedites threat recognition, and enables timely defense deployment. This proactive approach enhances cybersecurity strategies by minimizing threat active time and reducing the likelihood of illegal access and data breaches, fundamentally reshaping cybersecurity paradigms with AI’s speed, precision, and adaptability.
Endpoint security with AI
AI has transformed endpoint security in cybersecurity by exhibiting astounding precision and adaptability. Recent studies have demonstrated that its integration with endpoint security functions as a proactive defense is incredibly successful in preventing malware and thwarting hostile activities. It uses complex algorithms and machine learning to detect and remove viruses quickly. AI is particularly good at identifying normal and abnormal device activity patterns. Its effectiveness in spotting suspicious files and monitoring network activity and empirical studies demonstrate spotting patterns suggestive of malevolent intent. Recent research highlights AI’s versatility in managing changing threats, surpassing old signature-based techniques, and successfully thwarting zero-day exploits and creative malware that sidesteps conventional security procedures.
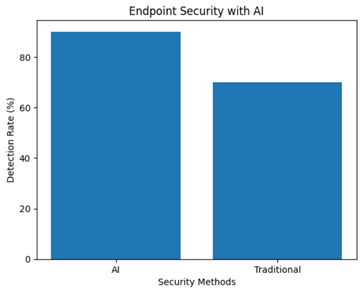
Predictive Analysis and Cybersecurity Intelligence
AI-powered predictive analysis is transforming cybersecurity by proactively identifying and averting potential intrusions. Its synergy highlights how important cybersecurity intelligence is to prevent such threats. AI agents examine large datasets for trends and irregularities with advanced algorithms and machine learning skills to anticipate new attack avenues. Recent studies demonstrate how AI-driven predictive analysis can give businesses a significant edge by fortifying defenses before threats manifest. Cybersecurity intelligence becomes crucial when it comes to providing timely insights into the strategies of threat actors and facilitating proactive modifications to security protocols, vulnerability patching, and preemptive countermeasures.
Challenges and Ethical Considerations
Artificial intelligence has revolutionary potential when combined with cybersecurity, but drawbacks and moral dilemmas exist. Realizing the complete potential of AI-powered security systems requires understanding and attention to these challenges.
Challenges
- Adversarial Attacks
One of the main issues with AI-driven cybersecurity is Vulnerability to hostile attacks. Artificial intelligence models can be tricked by skilled input data manipulators, jeopardizing the integrity of security protocols. Strong defenses are required in this never-ending game of cat and mouse to detect and prevent hostile attempts, which guarantees the dependability of AI-based systems. - Lack of Explain ability
One major obstacle that arises from the complexity of some AI algorithms, especially in deep learning models, is their unexplainability. It becomes difficult to comprehend the reasoning behind AI judgments, creating an algorithmic black box. This opacity makes it challenging to build openness and trust, which makes it tough to guarantee that AI systems can be understood and are subject to human scrutiny. - Data Privacy Concerns
The efficiency of AI depends on large datasets for training, but this dependence presents legitimate data privacy issues. The processing and storage of sensitive data raises privacy concerns in the context of cybersecurity. Regarding the moral application of AI-powered cybersecurity solutions, finding a balance between using data to improve security and protecting personal and organizational privacy becomes crucial. Encouraging user trust and adhering to privacy standards requires addressing these problems.
Ethical Considerations
- Biases in Training Data
Significant ethical issues arise from biases in the training data used by AI algorithms. When educated on biased datasets, AI algorithms have the potential to perpetuate preexisting prejudices. Given the possibility of skewed outcomes, this is particularly risky in the context of cybersecurity. Using mitigating strategies, diversifying data sources, and continuing oversight are critical to preserving justice and equity. If not, some groups might unfairly be singled out, and some threat vectors might go unrecognized. - Decision-Making Accountability
An intricate ethical landscape is introduced when deciding who is responsible for decisions made by AI systems. It is difficult to assign blame when mistakes or unexpected outcomes occur. Liability concerns surface, leading to a thorough investigation of who should bear responsibility for the results of cybersecurity decisions made using AI. Balancing algorithmic autonomy and human monitoring is imperative to ensure accountability and uphold ethical norms. - Unintended Consequences
The use of AI in cybersecurity raises the possibility of unforeseen repercussions. Organizations that rely too much on AI risk developing a false sense of security and becoming open to new attack vectors that AI systems must prepare to manage. This ethical concern highlights how crucial it is to keep a nuanced approach, acknowledge AI’s limitations and potential, and ensure that cybersecurity is approached with a human-centric perspective. It is essential to be thoroughly aware of the possible consequences to avoid relying too much on AI systems and to keep a flexible and proactive security posture.
Potential Risks
- Dependency and Overreliance
The risk of dependency and overreliance is a significant potential issue associated with integrating artificial intelligence into cybersecurity. While AI has unmatched potential, an over-dependence on technology surpassing human supervision could result in disastrous errors. It still takes human judgment and intervention to guarantee the efficacy and flexibility of security measures. Avoiding overreliance on AI requires carefully balancing utilizing its advantages and preserving human control. - Malicious Use of AI
Malicious actors may use AI technologies themselves, which are intended to improve cybersecurity. This creates a complicated dynamic in which attackers use AI algorithms to hone their tactics and duck capture. This ongoing game of cat and mouse in cyberspace highlights the necessity of continuing attention to detail and creativity in offensive and defensive cybersecurity tactics. Being ahead of the curve in this complex and dynamic environment requires anticipating and minimizing the possibility of malicious use of AI.
Case Studies
- Darktrace’s Autonomous Response in Action
Darktrace uses AI to improve cybersecurity, demonstrating the capabilities of its self-learning Cyber AI. In an actual case, the AI quickly identified and neutralized a fresh ransomware attack within the network of a global shipping company. The self-sufficient reaction, encompassing prompt isolation of impacted machines, underscores Darktrace’s inventiveness in anticipating and mitigating cyber hazards. - Cylance’s Proactive Threat Prevention
Cylance, a leader in AI-driven cybersecurity, exemplifies successful cyberattack prevention through its proactive strategy. Using artificial intelligence systems, Cylance identifies malicious intent in files before execution. In a specific instance, it thwarted a potential ransomware assault on a financial institution, showcasing the power of AI in preventing cybersecurity incidents through predictive analysis. - IBM watson for cybersecurity
The AI powers of IBM Watson excel in threat detection through the analysis of massive volumes of both structured and unstructured data. In a noteworthy instance, IBM Watson discovered a highly skilled cyberattack aimed at a multinational corporation. The AI engine not only found the hidden infection but also gave valuable details about the tactics used by the cybercriminals. This proactive intelligence highlights how AI transforms threat detection and provides actionable information for proactive cybersecurity protection. It enables business to fortify its defenses and avert a potential global compromise.
Future Trends in AI and Cyber Security
- Enhanced Threat Intelligence Integration
AI integration transforms cybersecurity by providing extensive threat details, enabling proactive identification and preparation for hazards. This strategic shift empowers organizations to stay ahead in the dynamic threat landscape. - Explainable AI for Trust and Compliance
Explainable AI ensures transparency in complex systems, crucial for compliance and trust in highly regulated sectors like banking and healthcare. It makes AI a transparent and understandable ally, meeting the demands of a society valuing data. - AI-Powered Autonomous Security Operations
AI-driven autonomous security operations streamline procedures, allowing cybersecurity experts to focus on strategic projects and proactive threat hunting. This mutually beneficial partnership reshapes the role of human knowledge, enhancing productivity against evolving cyber threats. - Quantum Computing Defense Strategies
AI develops advanced encryption techniques vital to cybersecurity defense against quantum computing. The objective is to build resilient defenses that can survive the unmatched computing capability of quantum computers. - Integration of AI with Human Expertise
The future of cybersecurity lies in the collaborative synergy of AI and human skills. AI acts as a strategic ally, enhancing cybersecurity professionals’ capabilities without replacing them. This partnership promotes a proactive approach, combining human intuition with AI’s analytical prowess to dynamically protect against cyber threats.
Conclusion
The convergence of artificial intelligence and cybersecurity signifies a paradigm leap in mitigating intricate cyber threats. The old reactive security strategy is changing due to AI’s proactive and adaptive capabilities, which are demonstrated in autonomous response systems, predictive analysis, and endpoint protection. Artificial intelligence has made remarkable progress in real-world applications despite obstacles such as adversarial attacks and ethical constraints. Emerging trends, including explainable AI, autonomous security operations, and joint human-AI knowledge, promise a robust cybersecurity framework. Responsible development and strategic cooperation ensure resilience against constantly changing digital threats.